Most artificial intelligence (AI) libraries and machine learning (ML) algorithms have been around for decades. However, easy access to these AI and ML libraries and large training data sets is relatively new.
The tech industry has come a long way since we worked on our first AI project for a client in 2009. Back then, we were a small startup team of less than 10 people. Even though 2021’s only beginning, Oursky saw inquiries about ML/AI projects more than double. Another testament to our own use of AI and ML is our very own FormX, our AI-powered data extraction and data capture tool. FormX helps with business process automation by transforming information from physical documents into structured digital data.
These got us to reflect on how AI has picked up in the past few years. The accessible libraries are freeing up entrepreneurial, indie, and freelance developers to think more about business ideas. Today’s technology landscape, too, are enabling developers to address business needs by creating new AI-powered applications and digital products.
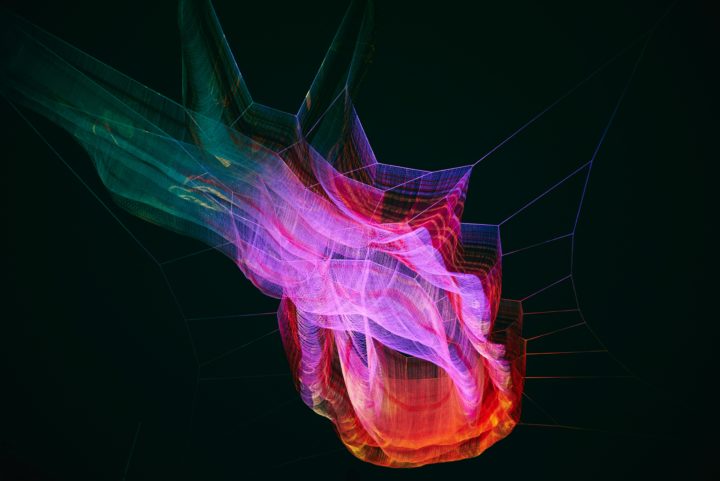
Humble Beginnings: How Oursky Got into AI and ML
Oursky’s first machine learning contract, back in 2009, was to develop a character recognition software to streamline identity verification. We experimented with neural networks, decision trees, and some optical character recognition (OCR) libraries. We ultimately went with neural network solutions because of their accuracy. Back then, we had to implement most of the algorithms from scratch since they were not really common.
The practical machine learning tools and techniques we experimented with have been around for decades. However, the tech industry, in general, was hampered by computing resources.
Some types of machine learning models/techniques, such as convolutional neural networks, have been applied since the 1980s. For example, LeNet-5, developed in 1998, helped some banks recognize handwritten numbers on cheques that were digitized as 32×32 pixel images. To work on more complex classification tasks, more and more convolutional layers are needed. At the time, this technique was restricted by the availability of processing power.
That all changed in the past couple of years. The hardware space has caught up, and it opened new opportunities for investment and commercialization.
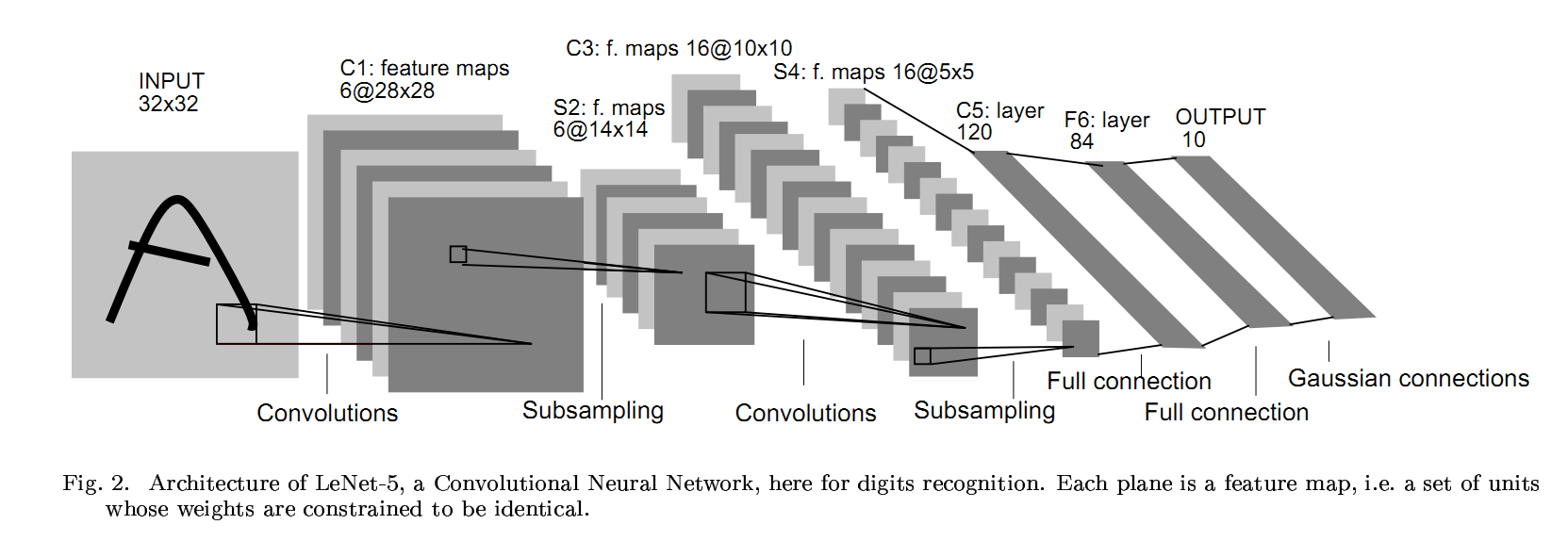
The Rise of AI: Why are AI and ML Now in Demand?
To put the potential of AI into perspective, investment in AI/ML in 2017 alone had already overtaken all of the combined investment in big data. Last year, AI adoption and investment further grew, with the pandemic forcing enterprises to fast-track their digital transformation. According to the Financier, investment will reach $37 billion by 2025, with China already leading the way as the second largest AI ecosystem after the US.
AI is picking up because the technology ecosystem has come together, including:
- The availability of open-source machine learning libraries like Microsoft’s CNTK, Theano, or Google’s TensorFlow
- Increased processing power (servers and consumer-grade processors) that helps with commercialization
- Cloud infrastructures that are now commonly used to store large quantities of business data (and thus have the potential to train machine learning models)
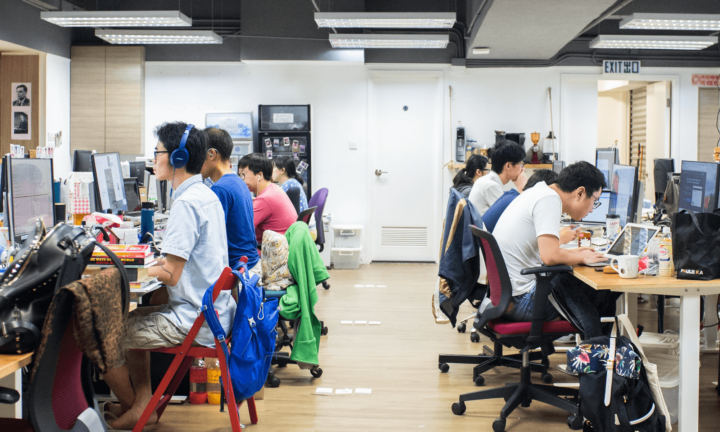
With practical machine learning tools and techniques more readily available, small teams or even indie and freelance developers can use these libraries to add AI-powered features to an application, rather than developing everything from scratch. This is something we’ve done for a side project that involved training a sequence-to-sequence chatbot in Cantonese from forum data.
Developers can now also use pre-trained models such as CNN libraries for Keras.io to avoid the computing cost of training every model from the ground up. You can even make use of free and cheap APIs or libraries for many common tasks, such as how we implemented face detection in AR applications in iOS (like for our client CheckCheckCin) and Android without writing any “low-level” code.
AI in Business: Companies Are Approaching AI and ML Differently from 5 Years Ago
When we first started 12 years ago, only a handful of clients dared to try adopting AI technologies. These clients usually had specific problems they wanted to solve, from generating chords from music files to identifying various zipper puller styles from an image and matching them through an inventory in a database.
Nowadays, Oursky has been getting more enquiries from IT or business departments and digital teams in large companies. They want to use AI, but they don’t have a clear picture of how different types of AI capabilities can be applied to their existing applications.
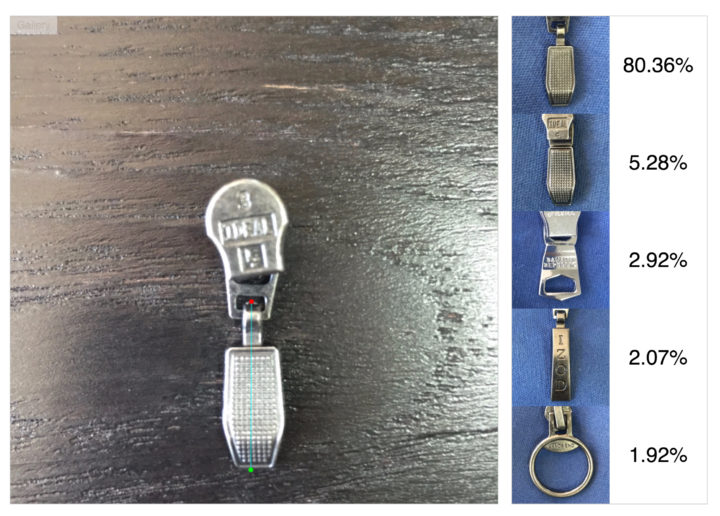
Many people also realize what AI can do but are not sure how to apply it to their business. While Oursky is not specialized in business consulting, we are now in a position to recommend AI solutions to clients that are sometimes more cost-effective for business operations in the long term. For example, businesses can think about target areas where AI can help in internal business operations (such as sorting data or detecting fraud) or improve front-line customer engagement (such as real-time product matching based on photos).
Current Trends in Artificial Intelligence
AI has come such a long way that businesses are using it more differently than ever. Here are just some of the emerging and current trends in artificial intelligence that software developers can take note of:
- Automating decision making and manual tasks such as in manufacturing and supply chain/production lines (i.e., robotic process automation)
- Delivering personalized digital customer experiences and recommendations in internet-of-things (IoT) devices and software (i.e., chatbots)
- Providing virtual and augmented reality (AR) capabilities in apps
- Creating different kinds of media — content, film, music, and games, to name a few
- Biometrics (i.e., facial recognition) and other recognition capabilities
Experimenting with Artificial Intelligence and Machine Learning Libraries
Machine learning research is also thriving. The extent of applications for neural networks was simply not yet fully understood 10 years ago. The generative adversarial network, which produces great visual images, is now having wider application when adapted to 3D model generation and the FinTech industry. The possibilities for other applications are endless! Transfer learning, which involves reapplying a trained AI model to a new context, became more widely understood and is now adopted for many applications, such as Google AutoML.
There are also many more AI-related products, usually in the form of APIs and libraries, that can be used for common applications so that developers don’t need to train a neural network for a specific purpose. An example is an app that we built to help clients record their team’s emotions and identify objects from images that leveraged robust public APIs and pre-trained networks.
Our team, for instance, tested the facial detection capability of our previously developed IoT door lock in 48 hours by leveraging open-source libraries. Only two of our colleagues worked on that Christmas hackathon project — that’s how accessible AI and ML has become!
Here are some top open-source AI frameworks, platforms, and tools to help developers get started:
- TensorFlow
- OpenNN
- IBM Watson
- scikit-learn
- Microsoft Cognitive Toolkit (CNTK)
- Caffe deep learning framework
- Torch
- Accord.NET
- Amazon SageMaker Neo
- Apache SystemsDS
- Apache Mahout
- Neuroph
- Deeplearning4j
- The OpenCog project
- Mycroft voice assistant
The Benefits of Artificial Intelligence and Understanding AI’s Use Cases
It’s important to understand the different parts of AI before thinking about adopting or applying it in the business. Virtual assistants, for example, have several different AI components that need to be implemented so it can provide a good service. For example, they need AI components to convert voice to text, process the text as natural language processing (NLP), perform an action if required, then convert the text back to speech. It may also have predictive abilities, which is also considered as machine learning.
Many AI components have already been incorporated into businesses. Everything from targeted ads on social media platforms; and item and price recommendations for sellers listing their items on Amazon; to stickers on Snapchat and Instagram are all AI-powered. Some exciting things are also happening on areas like algorithmic trading, which is based on sentiment with neural networks,; medical imaging like the Tencent-backed Voxelcloud; industrial robotics; Baidu’s Apollo for driverless cars; or a chatbot for banks in Singapore.
To fully reap the benefits of artificial intelligence in the business, you need to:
- Understand the particular use cases — that is, the problems you want to solve using AI and ML.
- Prepare quality data (and depending on the scope of your project, large amounts of it).
- Assess your infrastructures if they can handle the requirements.
On the other hand, using machine learning applications no longer requires hiring a large team of developers. Any developer with a buddy who has worked on AI projects can make use of open-source libraries to create new digital products and start experimenting (of course, we encourage open-sourcing).
Many startups and companies that provide AI services started out this way, and they’ve went on to become successful in their own right. Many companies are also investing and reaping the advantages of artificial intelligence for their business. With a flourishing AI industry, developers should strike while the iron is hot!
We’re excited to work on and grow with the global ML/AI development community! Feel free to ping us on Twitter or Facebook if you’ve got a great library to share! We also look forward to sharing new AI-powered products that we’re working on, so subscribe and stay tuned to our blog!
Oursky has a team of data scientists, AI and machine learning experts, and dedicated developers who work on AI projects for startups and multinational firms across different industries. Get in touch with us if you’re exploring AI solutions to your business, whether an AI-powered chatbot, prediction model, or recommendation engine. You can also learn more about Oursky’s AI solutions at skylab.ai.